Пов'язано з монетами
Калькулятор цін
Історія цін
Прогноз ціни
Технічний аналіз
Посібник з купівлі монет
Категорія криптовалют
Калькулятор прибутку

Ціна DriftDRIFT
Лістинг відбувся
КупитиВалюта котирування:
USD
Як ви ставитеся до Drift сьогодні?
Примітка. Ця інформація надається лише для ознайомлення.
Ціна Drift сьогодні
Ціна Drift в реальному часі становить $1.15 за (DRIFT / USD) за сьогодні з поточною ринковою капіталізацією $315.95M USD. Обсяг торгівлі за 24 години становить $28.42M USD. Ціна від DRIFT до USD оновлюється в режимі реального часу. Drift становить -0.02% за останні 24 години. Циркулююча пропозиція 273,751,900 .
Яка найвища ціна DRIFT?
Історичний максимум (ATH) DRIFT становить $2.65, зафіксований 2024-11-09.
Яка найнижча ціна DRIFT?
Історичний мінімум (ATL) DRIFT становить $0.1000, зафіксований 2024-05-16.
Прогноз для ціни Drift
Коли найкраще купувати DRIFT? Чи варто купувати або продавати DRIFT зараз?
Коли ви вирішуєте, купувати чи продавати DRIFT, необхідно спершу врахувати власну торгову стратегію. Торгова активність довгострокових і короткострокових трейдерів також буде відрізнятися. Технічний аналіз DRIFT від Bitget може надати вам орієнтир для торгівлі.
Згідно з Технічний аналіз DRIFT за 4 год., торговий сигнал — Продати.
Згідно з Технічний аналіз DRIFT за 1 день, торговий сигнал — Нейтрально.
Згідно з Технічний аналіз DRIFT за 1 тиждень, торговий сигнал — Купити.
Якою буде ціна DRIFT у 2026?
Ґрунтуючись на моделі прогнозування історичних показників DRIFT, ціна DRIFT може досягти $1.15 у $1.15 році.
Якою буде ціна DRIFT у 2031?
У 2031 ціна DRIFT може зрости на +19.00%. Прогнозується, що до кінця 2031 ціна DRIFT досягне $2.17, а сукупна ROI становитиме +85.15%.
Історія цін Drift (USD)
За останній рік ціна Drift зросла на +1053.43%. Найвища ціна DRIFT в USD минулого року була $2.65, а найнижча ціна DRIFT в USD — $0.1000.
ЧасЗміна ціни (%)
Найнижча ціна
Найвища ціна 
24h-0.02%$1.15$1.21
7d-15.13%$1.11$1.47
30d-10.05%$0.8791$1.54
90d+120.83%$0.3822$2.65
1y+1053.43%$0.1000$2.65
За весь час+1053.43%$0.1000(2024-05-16, 241 дні(в) тому )$2.65(2024-11-09, 64 дні(в) тому )
Ринкові дані Drift
Історія ринкової капіталізації Drift
Ринкова капіталізація
$315,947,184.73
-0.02%
Повністю розбавлена ринкова капіталізація
$1,154,136,977.35
-0.02%
Обсяг (24 г)
$28,415,719.57
-19.05%
Ринкові рейтинги
Показник обігу
27.00%
Обсяг за 24 г / Ринкова капіталізація
8.99%
Циркулююча пропозиція
273,751,900 DRIFT
Загальна пропозиція / Максимальна пропозиція
1,000,000,000 DRIFT
-- DRIFT
Ринок Drift
Утримання Drift за концентрацією
Кити
Інвестори
Рітейл
Адреси Drift за часом утримання
Холдери
Cruisers
Трейдери
Графік ціни coinInfo.name (12) у режимі реального часу
Оцінки Drift
Середні оцінки від спільноти
4.6
Цей контент призначено лише для інформаційних цілей.
DRIFT до місцевої валюти
1 DRIFT до MXN$23.911 DRIFT до GTQQ8.951 DRIFT до CLP$1,165.031 DRIFT до UGXSh4,286.151 DRIFT до HNLL29.481 DRIFT до ZARR22.061 DRIFT до TNDد.ت3.721 DRIFT до IQDع.د1,518.561 DRIFT до TWDNT$38.211 DRIFT до RSDдин.131.781 DRIFT до DOP$70.691 DRIFT до MYRRM5.191 DRIFT до GEL₾3.261 DRIFT до UYU$50.431 DRIFT до MADد.م.11.651 DRIFT до AZN₼1.961 DRIFT до OMRر.ع.0.441 DRIFT до KESSh150.061 DRIFT до SEKkr12.941 DRIFT до UAH₴49.02
- 1
- 2
- 3
- 4
- 5
Останнє оновлення 2025-01-12 11:29:08(UTC+0)
Як купити Drift(DRIFT)
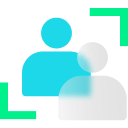
Вільно створіть акаунт на Bitget
Зареєструйтеся на Bitget, вказавши адресу електронної пошти/номер мобільного телефону, та створіть надійний пароль для захисту свого акаунта.
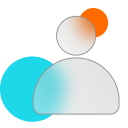
Верифікуйте свій акаунт
Верифікуйте свою особу, ввівши особисту інформацію та завантаживши дійсне посвідчення особи з фотографією.
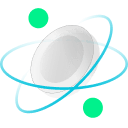
Купити Drift (DRIFT)
Скористайтеся різними способами оплати, щоб купити Drift на Bitget. Ми покажемо вам як.
ДокладнішеТоргуйте безстроковими фʼючерсами DRIFT
Після успішної реєстрації на Bitget і купівлі токенів USDT або DRIFT, ви можете почати торгувати деривативами, зокрема фʼючерсами на DRIFT, або скористатися маржинальною торгівлею, щоб збільшити свій дохід.
Актуальна ціна DRIFT дорівнює $1.15, а за 24 години ціна змінилася на -0.02%. Трейдери можуть заробляти на фʼючерсах DRIFT, відкриваючи лонг- або шорт-позиції.
Приєднуйтесь до копітрейдингу DRIFT, підписавшись на елітних трейдерів.
Після реєстрації на Bitget та успішної купівлі токенів USDT або DRIFT, ви також можете почати користуватися копітрейдингом, підписавшись на елітних трейдерів.
Drift новин
DRIFT перевищив 2 USDT, з 24-годинним зростанням на 332%
Bitget•2024-11-09 03:17
Токен Drift Protocol DRIFT перевищує $0,65, досягаючи рекордних висот
Bitget•2024-09-12 19:18
Нові лістинги на Bitget
Нові лістинги
Купити більше
Поширені запитання
Яка поточна ціна Drift?
Актуальна ціна Drift становить $1.15 за (DRIFT/USD), актуальна ринкова капіталізація становить $315,947,184.73 USD. Вартість Drift часто коливається через безперервну активність на криптовалютному ринку. Актуальну ціну Drift в режимі реального часу та дані на історії ви завжди можете переглянути на Bitget.
Який обсяг торгівлі Drift за 24 години?
За останні 24 години обсяг торгівлі Drift становить $28.42M.
Який історичний максимум Drift?
Історичний максимум Drift становить $2.65. Цей історичний максимум є найвищою ціною для Drift з моменту його запуску.
Чи можу я купити Drift на Bitget?
Так, Drift зараз можна придбати на централізованій біржі Bitget. Щоб отримати докладніші інструкції, перегляньте наш корисний посібник Як купити Drift protocol .
Чи можу я отримувати постійний дохід від інвестування в Drift?
Звичайно, Bitget забезпечує платформа для стратегічної торгівлі з розумними торговими ботами для автоматизації ваших угод і отримання прибутку.
Де можна купити Drift за найнижчою комісією?
Ми раді повідомити, що платформа для стратегічної торгівлі тепер доступний на Bitget. Bitget пропонує найкращі комісії за торгівлю та глибину ринку, щоб забезпечити прибутковість інвестицій для трейдерів.
Де можна купити Drift (DRIFT)?
Купуйте криптовалюту в застосунку Bitget
Щоб купити криптовалюту за допомогою кредитної картки або банківського переказу, вам потрібно зареєструватися. Це займе всього кілька хвилин.
Video section — Швидка верифікація, швидке здійснення угод
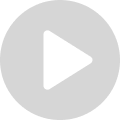
Як пройти верифікацію особи на Bitget та захистити себе від шахрайства
1. Увійдіть у свій акаунт Bitget.
2. Якщо ви ще не маєте акаунта на Bitget, перегляньте нашу інструкцію.
3. Наведіть курсор на значок вашого профілю, клацніть «Не верифікований», а потім «Верифікувати».
4. Оберіть країну або регіон, де ви отримали посвідчення особи, та тип посвідчення. Далі дотримуйтесь підказок на екрані.
5. Виберіть «Верифікація з мобільного» або «ПК».
6. Введіть свої дані, надішліть копію посвідчення особи та зробіть селфі.
7. Після цього подайте заявку, та все готово.
Інвестиції в криптовалюту, включаючи купівлю Drift онлайн через Bitget, підлягають ринковому ризику. Bitget надає вам прості та зручні способи купівлі Drift, і ми намагаємося максимально повно інформувати наших користувачів про кожну криптовалюту, яку ми пропонуємо на біржі. Однак ми не несемо відповідальності за результати, які можуть виникнути в результаті купівлі Drift. Ця сторінка та будь-яка інформація, що тут міститься, не є схваленням будь-якої конкретної криптовалюти.
Bitget Insights
BGUSER-SX0MCXKN
2дн.
Maitland of Al
The term "Maitland" is not a widely recognized term in the field of Artificial Intelligence (AI). However, I can provide some information on the concept of "maintland" or "maintenance land" in the context of AI.
In AI, maintenance land refers to the process of maintaining and updating AI systems to ensure they continue to function effectively and efficiently over time. This can include tasks such as:
1. _Data maintenance_: Ensuring that the data used to train and test AI models is accurate, complete, and up-to-date.
2. _Model maintenance_: Updating AI models to reflect changes in the underlying data or to improve their performance.
3. _Software maintenance_: Ensuring that the software used to deploy and manage AI systems is up-to-date and secure.
4. _Hardware maintenance_: Ensuring that the hardware used to support AI systems is functioning properly and is sufficient to meet the demands of the system.
Importance of Maitland in AI
The concept of maitland is important in AI because it ensures that AI systems continue to function effectively and efficiently over time. This can help to:
1. _Improve performance_: Regular maintenance can help to improve the performance of AI systems by ensuring that they are using the most up-to-date data and models.
2. _Reduce errors_: Maintenance can help to reduce errors and improve the accuracy of AI systems by ensuring that they are functioning correctly.
3. _Enhance security_: Maintenance can help to enhance the security of AI systems by ensuring that they are protected from cyber threats and that any vulnerabilities are patched.
4. _Increase trust_: Maintenance can help to increase trust in AI systems by ensuring that they are transparent, explainable, and fair.
Challenges of Maitland in AI
The challenges of maitland in AI include:
1. _Data quality_: Ensuring that the data used to train and test AI models is accurate, complete, and up-to-date can be a challenge.
2. _Model drift_: AI models can drift over time, which can affect their performance and accuracy.
3. _Software updates_: Ensuring that the software used to deploy and manage AI systems is up-to-date and secure can be a challenge.
4. _Hardware maintenance_: Ensuring that the hardware used to support AI systems is functioning properly and is sufficient to meet the demands of the system can be a challenge.
Best Practices for Maitland in AI
The best practices for maitland in AI include:
1. _Regular maintenance_: Regular maintenance is essential to ensure that AI systems continue to function effectively and efficiently over time.
2. _Data quality checks_: Data quality checks should be performed regularly to ensure that the data used to train and test AI models is accurate, complete, and up-to-date.
3. _Model monitoring_: AI models should be monitored regularly to ensure that they are performing as expected and to detect any drift or degradation.
4. _Software updates_: Software updates should be performed regularly to ensure that the software used to deploy and manage AI systems is up-to-date and secure.
5. _Hardware maintenance_: Hardware maintenance should be performed regularly to ensure that the hardware used to support AI systems is functioning properly and is sufficient to meet the demands of the system.$AL
AL0.00%
CYBER0.00%
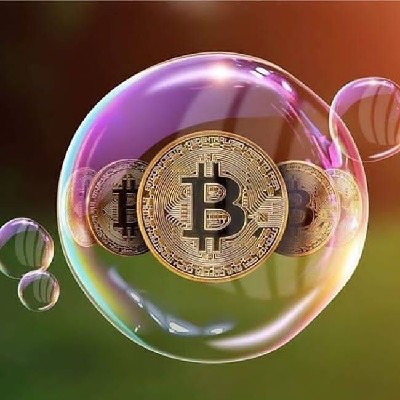
Crypto-Paris
2024/12/27 14:52
Deploying und Überwachung von Machine-Learning-Modellen
Deploying
1. Integrieren des Modells in den
Deploying und Überwachung von Machine-Learning-Modellen
Deploying
1. Integrieren des Modells in den Workflow
2. Bereitstellung der Ergebnisse für Benutzer/Entwickler
3. Konfiguration der Modellumgebung
Überwachung
1. *Modellleistung*: Überwachen von Genauigkeit und Leistung
2. *Data-Drift*: Erkennen von Datenveränderungen
3. *Modell-Degradation*: Überwachen der Modellleistung über die Zeit
4. *Benutzerfeedback*: Sammeln von Feedback für Verbesserungen
Erfolgskriterien
1. *Modellleistung*: Erforderliche Genauigkeit und Leistung erreicht
2. *Benutzerzufriedenheit*: Benutzer zufrieden mit Ergebnissen
3. *Stabilität*: Modell bleibt stabil und funktioniert ordnungsgemäß
Tools für Deploying und Überwachung
1. TensorFlow Serving
2. AWS SageMaker
3. Azure Machine Learning
4. Google Cloud AI Platform
5. Prometheus und Grafana für Überwachung
Best Practices
1. Kontinuierliche Integration und -lieferung
2. Automatisierte Tests
3. regelmäßige Überwachung und Analyse
4. Dokumentation und Kommunikation
5. kontinuierliche Verbesserung und Optimierung
CLOUD0.00%
DRIFT0.00%
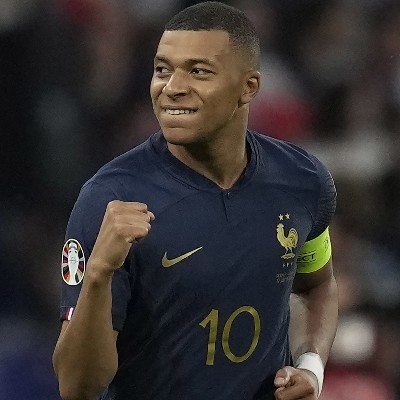
Kylian-mbappe
2024/12/27 14:25
Deploying und Überwachung von Machine-Learning-Modellen
Deploying
Das Deploying ist der letzte Schr
Deploying und Überwachung von Machine-Learning-Modellen
Deploying
Das Deploying ist der letzte Schritt eines Data-Analytics-Projekts. Hier werden die Machine-Learning-Modelle in den tatsächlichen Workflow integriert und die Ergebnisse für Benutzer oder Entwickler zugänglich gemacht.
Überwachung
Nach dem Deploying wird die Leistung des Modells überwacht, um Veränderungen wie Data-Drift oder Modell-Degradation zu erkennen. Wenn alles ordnungsgemäß funktioniert, kann das Projekt als erfolgreich betrachtet werden.
Schritte der Überwachung
1. *Modellleistung*: Überwachen der Modellleistung und -genauigkeit.
2. *Data-Drift*: Erkennen von Veränderungen in den Daten, die das Modell beeinflussen könnten.
3. *Modell-Degradation*: Überwachen der Modellleistung über die Zeit, um Degradation zu erkennen.
4. *Benutzerfeedback*: Sammeln von Feedback von Benutzern, um das Modell zu verbessern.
Erfolgskriterien
1. *Modellleistung*: Das Modell erreicht die erforderliche Genauigkeit und Leistung.
2. *Benutzerzufriedenheit*: Die Benutzer sind mit den Ergebnissen des Modells zufrieden.
3. *Stabilität*: Das Modell bleibt stabil und funktioniert ordnungsgemäß über die Zeit.
DRIFT0.00%
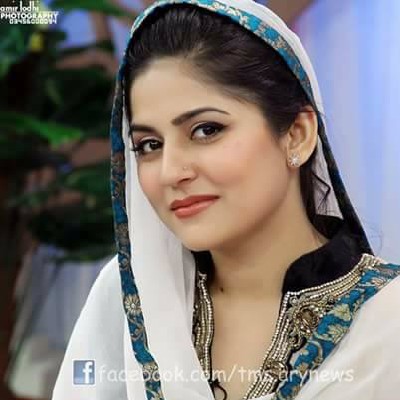
Sanam_Baloch
2024/12/27 14:07
The final stage of a data analytics project: deployment and monitoring. This is where the rubber meets the road, and the machine learning models are put into action.
During this stage, the analysts integrate the models into the actual workflow, making the outcomes available to users or developers. This is a critical step, as it ensures that the insights and predictions generated by the models are actionable and can drive business decisions.
Once the model is deployed, the analysts closely monitor its performance, watching for any changes that could impact its accuracy or effectiveness. This includes:
1. *Data drift*: Changes in the underlying data distribution that could affect the model's performance.
2. *Model degradation*: Decreases in the model's accuracy or performance over time.
3. *Concept drift*: Changes in the underlying relationships between variables that could impact the model's performance.
By monitoring the model's performance and addressing any issues that arise, the analysts can ensure that the project remains successful and continues to deliver value to the organization.
Some key activities during this stage include:
1. *Model serving*: Deploying the model in a production-ready environment.
2. *Monitoring and logging*: Tracking the model's performance and logging any issues or errors.
3. *Model maintenance*: Updating or retraining the model as needed to maintain its performance.
4. *Feedback loops*: Establishing processes to collect feedback from users or stakeholders and incorporating it into the model's development.
By following these steps, analysts can ensure that their data analytics project is not only successful but also sustainable and adaptable to changing business needs.
DRIFT0.00%
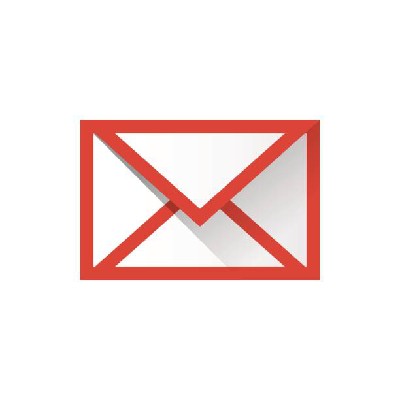
BGUSER-AEJ9PSGU
2024/12/27 13:58
Model Deployment and Monitoring
This is the last stage of a data analytics project. Here, analysts put the machine learning models into the actual workflow and make the outcomes available to users or developers. Once the model is deployed, they observe its performance for changes, like data drift, model degradation, etc. If everything appears operational, the project can be deemed successful.
DRIFT0.00%
Пов'язані активи
Популярні криптовалюти
Підбірка з 8 найкращих криптовалют за ринковою капіталізацією.
Нещодавно додано
Останні додані криптовалюти.
Порівнювана ринкова капіталізація
Серед усіх активів на Bitget ці 8 найближчі до Drift за ринковою капіталізацією.
