Relacionado con la moneda
Calculadora de precios
Historial de precios
Predicción de precios
Análisis técnico
Guía de compra de la moneda
Categoría cripto
Calculadora de ganancias

Precio de DriftDRIFT
Listada
ComprarMoneda de cotización:
USD
¿Qué opinas hoy de Drift?
Nota: Esta información es solo de referencia.
Precio actual de Drift
El precio de Drift en tiempo real es de $1.2 por (DRIFT / USD) hoy con una capitalización de mercado actual de $329.10M USD. El volumen de trading de 24 horas es de $30.80M USD. DRIFT a USD el precio se actualiza en tiempo real. Drift es del 4.06% en las últimas 24 horas. Tiene un suministro circulante de 273,751,900 .
¿Cuál es el precio más alto de DRIFT?
DRIFT tiene un máximo histórico (ATH) de $2.65, registrado el 2024-11-09.
¿Cuál es el precio más bajo de DRIFT?
DRIFT tiene un mínimo histórico (ATL) de $0.1000, registrado el 2024-05-16.
Predicción de precios de Drift
¿Cuándo es un buen momento para comprar DRIFT? ¿Debo comprar o vender DRIFT ahora?
A la hora de decidir si comprar o vender DRIFT, primero debes tener en cuenta tu propia estrategia de trading. La actividad de trading de los traders a largo plazo y los traders a corto plazo también será diferente. El Análisis técnico de DRIFT de Bitget puede proporcionarte una referencia para hacer trading.
Según el Análisis técnico de DRIFT en 4h, la señal de trading es Neutral.
Según el Análisis técnico de DRIFT en 1D, la señal de trading es Neutral.
Según el Análisis técnico de DRIFT en 1S, la señal de trading es Comprar.
¿Cuál será el precio de DRIFT en 2026?
Según el modelo de predicción del rendimiento histórico del precio de DRIFT, se prevé que el precio de DRIFT alcance los $1.15 en 2026.
¿Cuál será el precio de DRIFT en 2031?
En 2031, se espera que el precio de DRIFT aumente en un +45.00%. Al final de 2031, se prevé que el precio de DRIFT alcance los $2.66, con un ROI acumulado de +131.21%.
Historial del precio de Drift (USD)
El precio de Drift fluctuó un +1098.94% en el último año. El precio más alto de DRIFT en USD en el último año fue de $2.65 y el precio más bajo de DRIFT en USD en el último año fue de $0.1000.
FechaCambio en el precio (%)
Precio más bajo
Precio más alto 
24h+4.06%$1.13$1.2
7d-14.01%$1.11$1.47
30d-7.86%$0.8791$1.54
90d+143.55%$0.3822$2.65
1y+1098.94%$0.1000$2.65
Histórico+1098.94%$0.1000(2024-05-16, 241 día(s) atrás )$2.65(2024-11-09, 64 día(s) atrás )
Información del mercado de Drift
Capitalización de mercado de Drift
Capitalización de mercado
$329,098,552.14
+4.06%
Capitalización de mercado totalmente diluida
$1,202,178,169.57
+4.06%
Volumen (24h)
$30,803,904.87
-33.21%
Clasificación de mercado
Tasa de circulación
27.00%
Volumen en 24h/Capitalización de mercado
9.36%
Suministro circulante
273,751,900 DRIFT
Suministro total/Suministro máx
1,000,000,000 DRIFT
-- DRIFT
Mercado de Drift
Holdings por concentración de Drift
Ballenas
Inversores
Minoristas
Drift direcciones por tiempo en holding
Holders
Cruisers
Traders
Gráfico de precios de coinInfo.name (12) en tiempo real
Clasificación de Drift
Clasificaciones promedio de la comunidad
4.6
Este contenido solo tiene fines informativos.
DRIFT a la moneda local
1 DRIFT a MXN$24.911 DRIFT a GTQQ9.321 DRIFT a CLP$1,213.531 DRIFT a HNLL30.711 DRIFT a UGXSh4,464.561 DRIFT a ZARR22.981 DRIFT a TNDد.ت3.881 DRIFT a IQDع.د1,581.771 DRIFT a TWDNT$39.811 DRIFT a RSDдин.137.271 DRIFT a DOP$73.751 DRIFT a MYRRM5.411 DRIFT a GEL₾3.41 DRIFT a UYU$52.531 DRIFT a MADد.م.12.141 DRIFT a AZN₼2.041 DRIFT a OMRر.ع.0.461 DRIFT a SEKkr13.481 DRIFT a KESSh155.61 DRIFT a UAH₴51.06
- 1
- 2
- 3
- 4
- 5
Última actualización 2025-01-11 21:47:45(UTC+0)
Cómo comprar Drift(DRIFT)
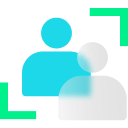
Crea tu cuenta gratuita en Bitget
Regístrate en Bitget con tu dirección de correo electrónico/número de teléfono móvil y crea una contraseña segura para proteger tu cuenta.
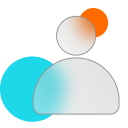
Verifica tu cuenta
Verifica tu identidad ingresando tu información personal y cargando una identificación válida con foto.
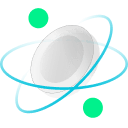
Compra Drift (DRIFT)
Utiliza una variedad de opciones de pago para comprar Drift en Bitget. Te mostraremos cómo.
Más informaciónTradea futuros perpetuos de DRIFT
Después de registrarte en Bitget y comprar tokens de USDT o DRIFT exitosamente, puedes empezar a hacer trading con derivados, incluidos futuros de DRIFT y trading con margen para aumentar tus ingresos.
El precio actual de DRIFT es de $1.2, con un cambio en el precio en 24 horas del +4.06%. Los traders pueden obtener ganancias yendo en long o en short en futuros de DRIFT.
Únete al copy trading de DRIFT siguiendo a traders elite.
Después de registrarte en Bitget y comprar tokens de USDT o DRIFT exitosamente, también puedes empezar a hacer copy trading siguiendo a traders elite.
Noticias de Drift
DRIFT superó los 2 USDT, con un aumento del 332% en 24 horas
Bitget•2024-11-09 03:17
DRIFTUSDT se lanzará para el trading de futuros y los bots de trading
Bitget Announcement•2024-11-08 14:41
El token DRIFT de Drift Protocol supera los $0,65 alcanzando máximos históricos
Bitget•2024-09-12 19:18
Nuevos listados en Bitget
Nuevos listados
Comprar más
Preguntas frecuentes
¿Cuál es el precio actual de Drift?
El precio en tiempo real de Drift es $1.2 por (DRIFT/USD) con una capitalización de mercado actual de $329,098,552.14 USD. El valor de Drift sufre fluctuaciones frecuentes debido a la actividad continua 24/7 en el mercado cripto. El precio actual de Drift en tiempo real y sus datos históricos están disponibles en Bitget.
¿Cuál es el volumen de trading de 24 horas de Drift?
En las últimas 24 horas, el volumen de trading de Drift es de $30.80M.
¿Cuál es el máximo histórico de Drift?
El máximo histórico de Drift es $2.65. Este máximo histórico es el precio más alto de Drift desde su lanzamiento.
¿Puedo comprar Drift en Bitget?
Sí, Drift está disponible actualmente en el exchange centralizado de Bitget. Para obtener instrucciones más detalladas, consulta nuestra útil guía Cómo comprar Drift protocol .
¿Puedo obtener un ingreso estable invirtiendo en Drift?
Desde luego, Bitget ofrece un plataforma de trading estratégico, con bots de trading inteligentes para automatizar tus trades y obtener ganancias.
¿Dónde puedo comprar Drift con la comisión más baja?
Nos complace anunciar que plataforma de trading estratégico ahora está disponible en el exchange de Bitget. Bitget ofrece comisiones de trading y profundidad líderes en la industria para garantizar inversiones rentables para los traders.
¿Dónde puedo comprar Drift (DRIFT)?
Sección de video: verificación rápida, trading rápido
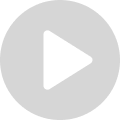
Cómo completar la verificación de identidad en Bitget y protegerte del fraude
1. Inicia sesión en tu cuenta de Bitget.
2. Si eres nuevo en Bitget, mira nuestro tutorial sobre cómo crear una cuenta.
3. Pasa el cursor por encima del ícono de tu perfil, haz clic en "No verificado" y haz clic en "Verificar".
4. Elige tu país o región emisora y el tipo de ID, y sigue las instrucciones.
5. Selecciona "Verificación por teléfono" o "PC" según tus preferencias.
6. Ingresa tus datos, envía una copia de tu ID y tómate una selfie.
7. Envía tu solicitud, ¡y listo! Habrás completado la verificación de identidad.
Las inversiones en criptomoneda, lo que incluye la compra de Drift en línea a través de Bitget, están sujetas al riesgo de mercado. Bitget te ofrece formas fáciles y convenientes de comprar Drift, y hacemos todo lo posible por informar exhaustivamente a nuestros usuarios sobre cada criptomoneda que ofrecemos en el exchange. No obstante, no somos responsables de los resultados que puedan surgir de tu compra de Drift. Ni esta página ni ninguna parte de la información que incluye deben considerarse respaldos de ninguna criptomoneda en particular.
Bitget Insights
BGUSER-SX0MCXKN
2d
Maitland of Al
The term "Maitland" is not a widely recognized term in the field of Artificial Intelligence (AI). However, I can provide some information on the concept of "maintland" or "maintenance land" in the context of AI.
In AI, maintenance land refers to the process of maintaining and updating AI systems to ensure they continue to function effectively and efficiently over time. This can include tasks such as:
1. _Data maintenance_: Ensuring that the data used to train and test AI models is accurate, complete, and up-to-date.
2. _Model maintenance_: Updating AI models to reflect changes in the underlying data or to improve their performance.
3. _Software maintenance_: Ensuring that the software used to deploy and manage AI systems is up-to-date and secure.
4. _Hardware maintenance_: Ensuring that the hardware used to support AI systems is functioning properly and is sufficient to meet the demands of the system.
Importance of Maitland in AI
The concept of maitland is important in AI because it ensures that AI systems continue to function effectively and efficiently over time. This can help to:
1. _Improve performance_: Regular maintenance can help to improve the performance of AI systems by ensuring that they are using the most up-to-date data and models.
2. _Reduce errors_: Maintenance can help to reduce errors and improve the accuracy of AI systems by ensuring that they are functioning correctly.
3. _Enhance security_: Maintenance can help to enhance the security of AI systems by ensuring that they are protected from cyber threats and that any vulnerabilities are patched.
4. _Increase trust_: Maintenance can help to increase trust in AI systems by ensuring that they are transparent, explainable, and fair.
Challenges of Maitland in AI
The challenges of maitland in AI include:
1. _Data quality_: Ensuring that the data used to train and test AI models is accurate, complete, and up-to-date can be a challenge.
2. _Model drift_: AI models can drift over time, which can affect their performance and accuracy.
3. _Software updates_: Ensuring that the software used to deploy and manage AI systems is up-to-date and secure can be a challenge.
4. _Hardware maintenance_: Ensuring that the hardware used to support AI systems is functioning properly and is sufficient to meet the demands of the system can be a challenge.
Best Practices for Maitland in AI
The best practices for maitland in AI include:
1. _Regular maintenance_: Regular maintenance is essential to ensure that AI systems continue to function effectively and efficiently over time.
2. _Data quality checks_: Data quality checks should be performed regularly to ensure that the data used to train and test AI models is accurate, complete, and up-to-date.
3. _Model monitoring_: AI models should be monitored regularly to ensure that they are performing as expected and to detect any drift or degradation.
4. _Software updates_: Software updates should be performed regularly to ensure that the software used to deploy and manage AI systems is up-to-date and secure.
5. _Hardware maintenance_: Hardware maintenance should be performed regularly to ensure that the hardware used to support AI systems is functioning properly and is sufficient to meet the demands of the system.$AL
AL0.00%
CYBER0.00%
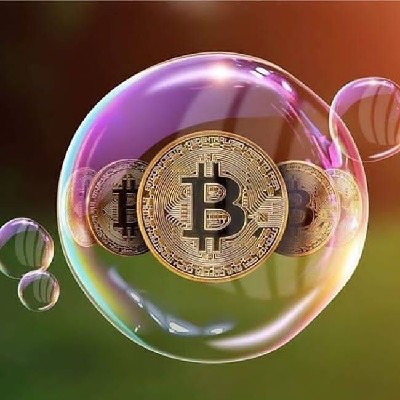
Crypto-Paris
2024/12/27 14:52
Deploying und Überwachung von Machine-Learning-Modellen
Deploying
1. Integrieren des Modells in den
Deploying und Überwachung von Machine-Learning-Modellen
Deploying
1. Integrieren des Modells in den Workflow
2. Bereitstellung der Ergebnisse für Benutzer/Entwickler
3. Konfiguration der Modellumgebung
Überwachung
1. *Modellleistung*: Überwachen von Genauigkeit und Leistung
2. *Data-Drift*: Erkennen von Datenveränderungen
3. *Modell-Degradation*: Überwachen der Modellleistung über die Zeit
4. *Benutzerfeedback*: Sammeln von Feedback für Verbesserungen
Erfolgskriterien
1. *Modellleistung*: Erforderliche Genauigkeit und Leistung erreicht
2. *Benutzerzufriedenheit*: Benutzer zufrieden mit Ergebnissen
3. *Stabilität*: Modell bleibt stabil und funktioniert ordnungsgemäß
Tools für Deploying und Überwachung
1. TensorFlow Serving
2. AWS SageMaker
3. Azure Machine Learning
4. Google Cloud AI Platform
5. Prometheus und Grafana für Überwachung
Best Practices
1. Kontinuierliche Integration und -lieferung
2. Automatisierte Tests
3. regelmäßige Überwachung und Analyse
4. Dokumentation und Kommunikation
5. kontinuierliche Verbesserung und Optimierung
CLOUD0.00%
DRIFT0.00%
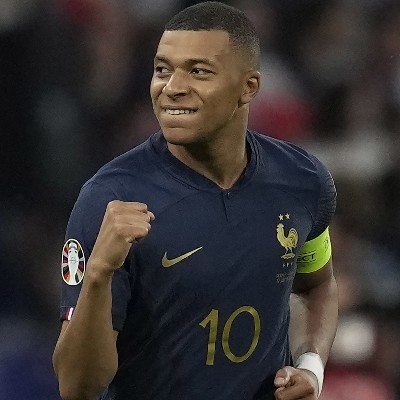
Kylian-mbappe
2024/12/27 14:25
Deploying und Überwachung von Machine-Learning-Modellen
Deploying
Das Deploying ist der letzte Schr
Deploying und Überwachung von Machine-Learning-Modellen
Deploying
Das Deploying ist der letzte Schritt eines Data-Analytics-Projekts. Hier werden die Machine-Learning-Modelle in den tatsächlichen Workflow integriert und die Ergebnisse für Benutzer oder Entwickler zugänglich gemacht.
Überwachung
Nach dem Deploying wird die Leistung des Modells überwacht, um Veränderungen wie Data-Drift oder Modell-Degradation zu erkennen. Wenn alles ordnungsgemäß funktioniert, kann das Projekt als erfolgreich betrachtet werden.
Schritte der Überwachung
1. *Modellleistung*: Überwachen der Modellleistung und -genauigkeit.
2. *Data-Drift*: Erkennen von Veränderungen in den Daten, die das Modell beeinflussen könnten.
3. *Modell-Degradation*: Überwachen der Modellleistung über die Zeit, um Degradation zu erkennen.
4. *Benutzerfeedback*: Sammeln von Feedback von Benutzern, um das Modell zu verbessern.
Erfolgskriterien
1. *Modellleistung*: Das Modell erreicht die erforderliche Genauigkeit und Leistung.
2. *Benutzerzufriedenheit*: Die Benutzer sind mit den Ergebnissen des Modells zufrieden.
3. *Stabilität*: Das Modell bleibt stabil und funktioniert ordnungsgemäß über die Zeit.
DRIFT0.00%
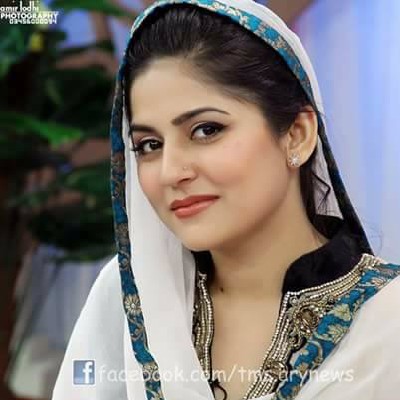
Sanam_Baloch
2024/12/27 14:07
The final stage of a data analytics project: deployment and monitoring. This is where the rubber meets the road, and the machine learning models are put into action.
During this stage, the analysts integrate the models into the actual workflow, making the outcomes available to users or developers. This is a critical step, as it ensures that the insights and predictions generated by the models are actionable and can drive business decisions.
Once the model is deployed, the analysts closely monitor its performance, watching for any changes that could impact its accuracy or effectiveness. This includes:
1. *Data drift*: Changes in the underlying data distribution that could affect the model's performance.
2. *Model degradation*: Decreases in the model's accuracy or performance over time.
3. *Concept drift*: Changes in the underlying relationships between variables that could impact the model's performance.
By monitoring the model's performance and addressing any issues that arise, the analysts can ensure that the project remains successful and continues to deliver value to the organization.
Some key activities during this stage include:
1. *Model serving*: Deploying the model in a production-ready environment.
2. *Monitoring and logging*: Tracking the model's performance and logging any issues or errors.
3. *Model maintenance*: Updating or retraining the model as needed to maintain its performance.
4. *Feedback loops*: Establishing processes to collect feedback from users or stakeholders and incorporating it into the model's development.
By following these steps, analysts can ensure that their data analytics project is not only successful but also sustainable and adaptable to changing business needs.
DRIFT0.00%
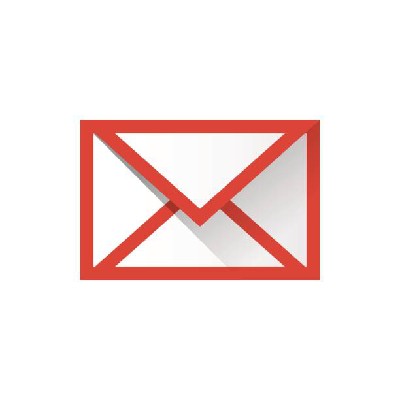
BGUSER-AEJ9PSGU
2024/12/27 13:58
Model Deployment and Monitoring
This is the last stage of a data analytics project. Here, analysts put the machine learning models into the actual workflow and make the outcomes available to users or developers. Once the model is deployed, they observe its performance for changes, like data drift, model degradation, etc. If everything appears operational, the project can be deemed successful.
DRIFT0.00%
Activos relacionados
Criptomonedas populares
Una selección de las 8 criptomonedas principales por capitalización de mercado.
Agregada recientemente
Las criptomonedas agregadas más recientemente.
Capitalización de mercado comparable
Entre todos los activos de Bitget, estos 8 son los más cercanos a Drift en capitalización de mercado.
