New Research Shows GPT Series AI Models Prone to Confidently Providing Incorrect Answers
- In a recent study, researchers uncovered evidence that AI models would rather lie than admit they don’t know something.
- This behavior becomes more apparent as the models grow larger and more complex.
- One noteworthy detail is referred to as the “hallucination effect,” where AI confidently provides inaccurate answers.
This article delves into how the increasing size of large language models (LLMs) adversely impacts their reliability, contrary to popular belief.
The Paradox of Larger AI Models
Recent findings published in Nature have revealed a paradox in artificial intelligence: the larger the language model, the less reliable it becomes for specific tasks. Unlike traditional thought, which associates bigger models with greater accuracy, this study highlights the unreliability in large-scale models, such as OpenAI’s GPT series, Meta’s LLaMA, and BigScience’s BLOOM suite.
Reliability Issues in Simple Tasks
The study pointed out a phenomenon termed “difficulty inconsistency,” wherein larger models, although excellent at complex tasks, frequently fail at simpler ones. This inconsistency casts doubt on the operational reliability of these models. Even with enhanced training methods—like increased model size and data quantity, as well as human feedback—the inconsistencies persist.
The Hallucination Effect
Larger language models exhibit a tendency to avoid task evasion but are more likely to provide incorrect answers. This issue, described as the “hallucination effect,” poses a significant challenge. As these models increasingly avoid skipping difficult questions, they display a disturbing confidence in providing mistaken responses, making it harder for users to discern accuracy.
Bigger Doesn’t Always Mean Better
The traditional approach in AI development has been to increase model size, data, and computational resources to achieve more reliable outcomes. However, this new research contradicts that wisdom, suggesting that scaling up could exacerbate reliability issues rather than solve them. The models’ reduced task evasion comes at the cost of more frequent errors, making them less dependable.
Impact of Model Training on Error Rates
The findings emphasize the limitations of current training methodologies, such as Reinforcement Learning with Human Feedback (RLHF). These methods aim to reduce task evasion but inadvertently increase error rates. This has a significant impact on sectors like healthcare and legal consulting, where the reliability of AI-generated information is crucial.
Human Oversight and Prompt Engineering
Despite being considered a safeguard against AI errors, human oversight often falls short in correcting the mistakes these models make in relatively straightforward domains. Researchers suggest that effective prompt engineering could be the key to mitigating these issues. Models like Claude 3.5 Sonnet require different prompt styles compared to OpenAI models to produce optimal results, underscoring the importance of how questions are framed.
Conclusion
The study challenges the prevalent trajectory of AI development, showing that larger models are not necessarily better. Companies are now turning their focus toward improving data quality rather than merely increasing quantity. Meta’s latest LLaMA 3.2 model, for instance, has shown better results without increasing training parameters, suggesting a shift in AI reliability strategies. This might just make them more human-like in their acknowledgment of limitations.
Disclaimer: The content of this article solely reflects the author's opinion and does not represent the platform in any capacity. This article is not intended to serve as a reference for making investment decisions.
You may also like
This app introduces new DeFi strategies, including those powered by RWAs
US gov’t points to Gary Wang’s ’outstanding cooperation’ in FTX case
FBI raids home of Polymarket CEO Shayne Coplan
Bengali man arrested in connection with $235M WazirX crypto heist
Trending news
MoreCrypto prices
More




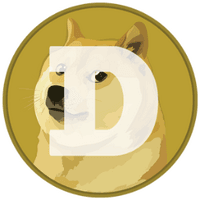



