Bitget: ¡En el Top 4 por volumen de trading diario global!
Cuota de mercado de BTC61.32%
Nuevos listados en Bitget : Pi Network
BTC/USDT$84300.01 (+0.92%)Índice de miedo y codicia34(Miedo)
Índice de la temporada de altcoins:0(Temporada de Bitcoin)
Monedas listadas en el pre-mercadoPAWS,WCTFlujo neto total de ETF en spot de Bitcoin -$60.6M (1d); +$218.9M (7d). Paquete de obsequios de bienvenida para nuevos usuarios por valor de 6.200 USDT.Reclamar ahora
Tradea en cualquier momento y lugar con la app de Bitget. Descargar ahora
Bitget: ¡En el Top 4 por volumen de trading diario global!
Cuota de mercado de BTC61.32%
Nuevos listados en Bitget : Pi Network
BTC/USDT$84300.01 (+0.92%)Índice de miedo y codicia34(Miedo)
Índice de la temporada de altcoins:0(Temporada de Bitcoin)
Monedas listadas en el pre-mercadoPAWS,WCTFlujo neto total de ETF en spot de Bitcoin -$60.6M (1d); +$218.9M (7d). Paquete de obsequios de bienvenida para nuevos usuarios por valor de 6.200 USDT.Reclamar ahora
Tradea en cualquier momento y lugar con la app de Bitget. Descargar ahora
Bitget: ¡En el Top 4 por volumen de trading diario global!
Cuota de mercado de BTC61.32%
Nuevos listados en Bitget : Pi Network
BTC/USDT$84300.01 (+0.92%)Índice de miedo y codicia34(Miedo)
Índice de la temporada de altcoins:0(Temporada de Bitcoin)
Monedas listadas en el pre-mercadoPAWS,WCTFlujo neto total de ETF en spot de Bitcoin -$60.6M (1d); +$218.9M (7d). Paquete de obsequios de bienvenida para nuevos usuarios por valor de 6.200 USDT.Reclamar ahora
Tradea en cualquier momento y lugar con la app de Bitget. Descargar ahora
Relacionado con la moneda
Calculadora de precios
Historial de precios
Predicción de precios
Análisis técnico
Guía de compra de la moneda
Categoría cripto
Calculadora de ganancias
Relacionado con el trading
Comprar/vender
Depositar/retirar
Spot
Margen
Futuros USDT-M
Futuros Coin-M
Bots de trading
Copy trading
Earn
Pre-mercado
Actualizaciones de la moneda
Noticias de Bonk on ETH
Alerta de Bonk on ETH
Calendario de eventos
ICO
Airdrop

Precio de Bonk on ETHBONK
No listado
Moneda de cotización:
EUR
Los datos proceden de proveedores externos. Esta página y la información proporcionada no respaldan ninguna criptomoneda específica. ¿Quieres tradear monedas listadas? Haz clic aquí
€0.{9}5038-1.49%1D
Gráfico de precios
Última actualización el 2025-04-01 14:55:28(UTC+0)
Capitalización de mercado:--
Capitalización de mercado totalmente diluida:--
Volumen (24h):--
Volumen en 24h/Capitalización de mercado:0.00%
Máximo 24h:€0.{9}4908
Mínimo 24h:€0.{9}4802
Máximo histórico:€0.{7}5453
Mínimo histórico:€0.{9}4802
Suministro circulante:-- BONK
Suministro total:
100,000,000,000,000BONK
Tasa de circulación:0.00%
Suministro máx.:
--BONK
Precio en BTC:0.{14}6519 BTC
Precio en ETH:0.{6}1226 ETH
Precio en la capitalización de mercado de BTC:
--
Precio en la capitalización de mercado de ETH:
--
Contratos:
0x4fba...edc9b64(Ethereum)
Más
¿Qué opinas hoy de Bonk on ETH?
Nota: Esta información es solo de referencia.
Precio actual de Bonk on ETH
El precio de Bonk on ETH en tiempo real es de €0.{9}5038 por (BONK / EUR) hoy con una capitalización de mercado actual de €0.00 EUR. El volumen de trading de 24 horas es de €0.00 EUR. BONK a EUR el precio se actualiza en tiempo real. Bonk on ETH es del -1.49% en las últimas 24 horas. Tiene un suministro circulante de 0 .
¿Cuál es el precio más alto de BONK?
BONK tiene un máximo histórico (ATH) de €0.{7}5453, registrado el 2024-05-11.
¿Cuál es el precio más bajo de BONK?
BONK tiene un mínimo histórico (ATL) de €0.{9}4802, registrado el 2025-03-30.
Predicción de precios de Bonk on ETH
¿Cuál será el precio de BONK en 2026?
Según el modelo de predicción del rendimiento histórico del precio de BONK, se prevé que el precio de BONK alcance los €0.{9}6415 en 2026.
¿Cuál será el precio de BONK en 2031?
En 2031, se espera que el precio de BONK aumente en un +40.00%. Al final de 2031, se prevé que el precio de BONK alcance los €0.{8}1067, con un ROI acumulado de +117.66%.
Historial del precio de Bonk on ETH (EUR)
El precio de Bonk on ETH fluctuó un -96.32% en el último año. El precio más alto de en EUR en el último año fue de €0.{7}5453 y el precio más bajo de en EUR en el último año fue de €0.{9}4802.
FechaCambio en el precio (%)
Precio más bajo
Precio más alto 
24h-1.49%€0.{9}4802€0.{9}4908
7d-10.32%€0.{9}4802€0.{9}5512
30d-8.15%€0.{9}4802€0.{9}6873
90d-81.50%€0.{9}4802€0.{8}2670
1y-96.32%€0.{9}4802€0.{7}5453
Histórico-96.32%€0.{9}4802(2025-03-30, Ayer )€0.{7}5453(2024-05-11, 325 día(s) atrás )
Información del mercado de Bonk on ETH
Capitalización de mercado de Bonk on ETH
Capitalización de mercado
--
Capitalización de mercado totalmente diluida
€50,379
Clasificación de mercado
Holdings por concentración de Bonk on ETH
Ballenas
Inversores
Minoristas
Bonk on ETH direcciones por tiempo en holding
Holders
Cruisers
Traders
Gráfico de precios de coinInfo.name (12) en tiempo real
Clasificación de Bonk on ETH
Clasificaciones promedio de la comunidad
4.4
Este contenido solo tiene fines informativos.
BONK a la moneda local
1 BONK a MXN$01 BONK a GTQQ01 BONK a CLP$01 BONK a UGXSh01 BONK a HNLL01 BONK a ZARR01 BONK a TNDد.ت01 BONK a IQDع.د01 BONK a TWDNT$01 BONK a RSDдин.01 BONK a DOP$01 BONK a MYRRM01 BONK a GEL₾01 BONK a UYU$01 BONK a MADد.م.01 BONK a AZN₼01 BONK a OMRر.ع.01 BONK a KESSh01 BONK a SEKkr01 BONK a UAH₴0
- 1
- 2
- 3
- 4
- 5
Última actualización el 2025-04-01 14:55:28(UTC+0)
Noticias de Bonk on ETH
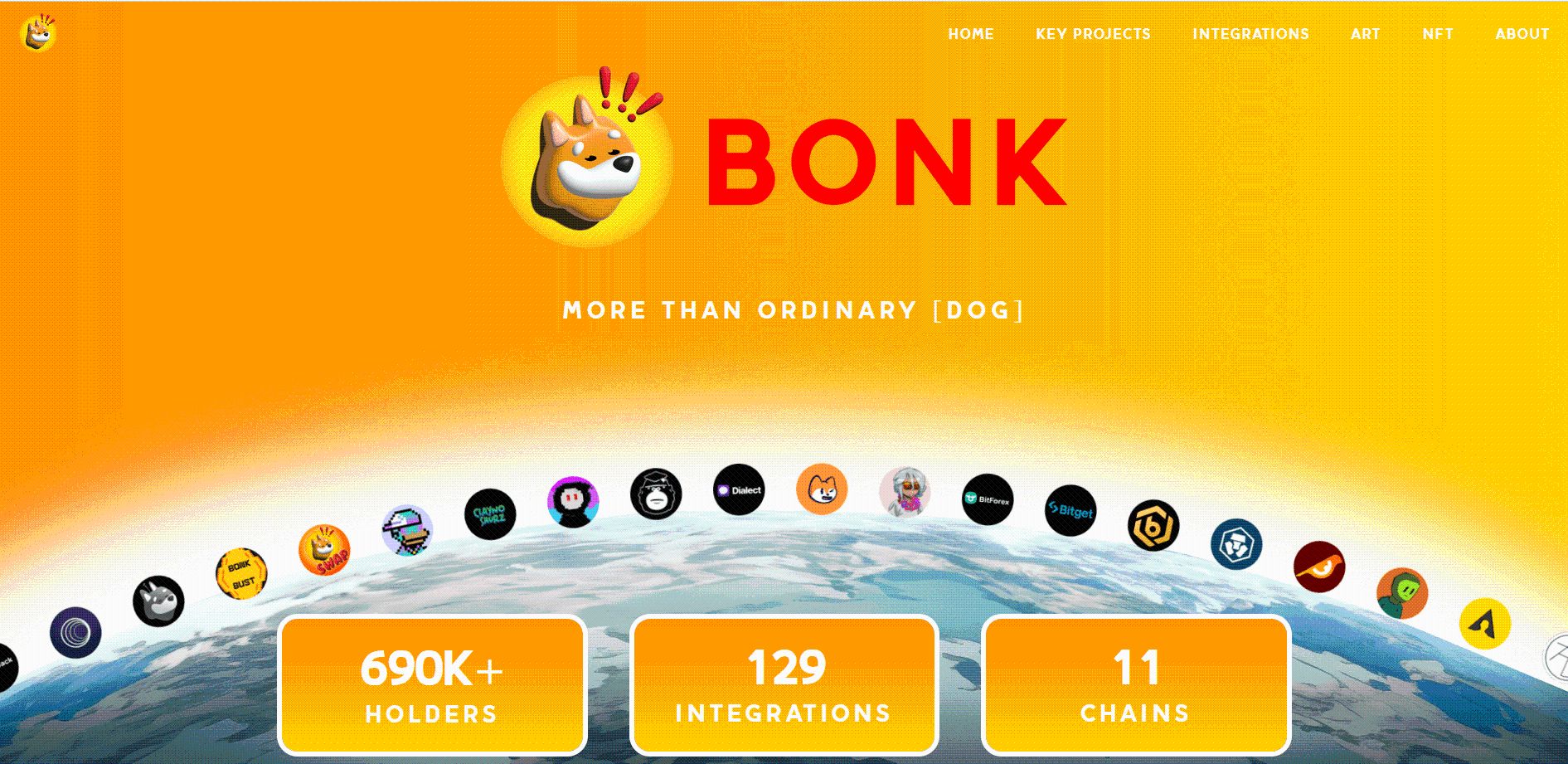
BONK, la memecoin con sede en Solana, ha adquirido Exchange Art
Coinjournal•2025-03-28 01:22
BONK anuncia la adquisición de la plataforma de mercado de arte Exchange
Bitget•2025-03-27 14:44
Predicción de precios de Bonk: ¿dónde tomará esta nueva señal de compra la Memecoin?
CryptoNewsNet•2025-03-22 15:33
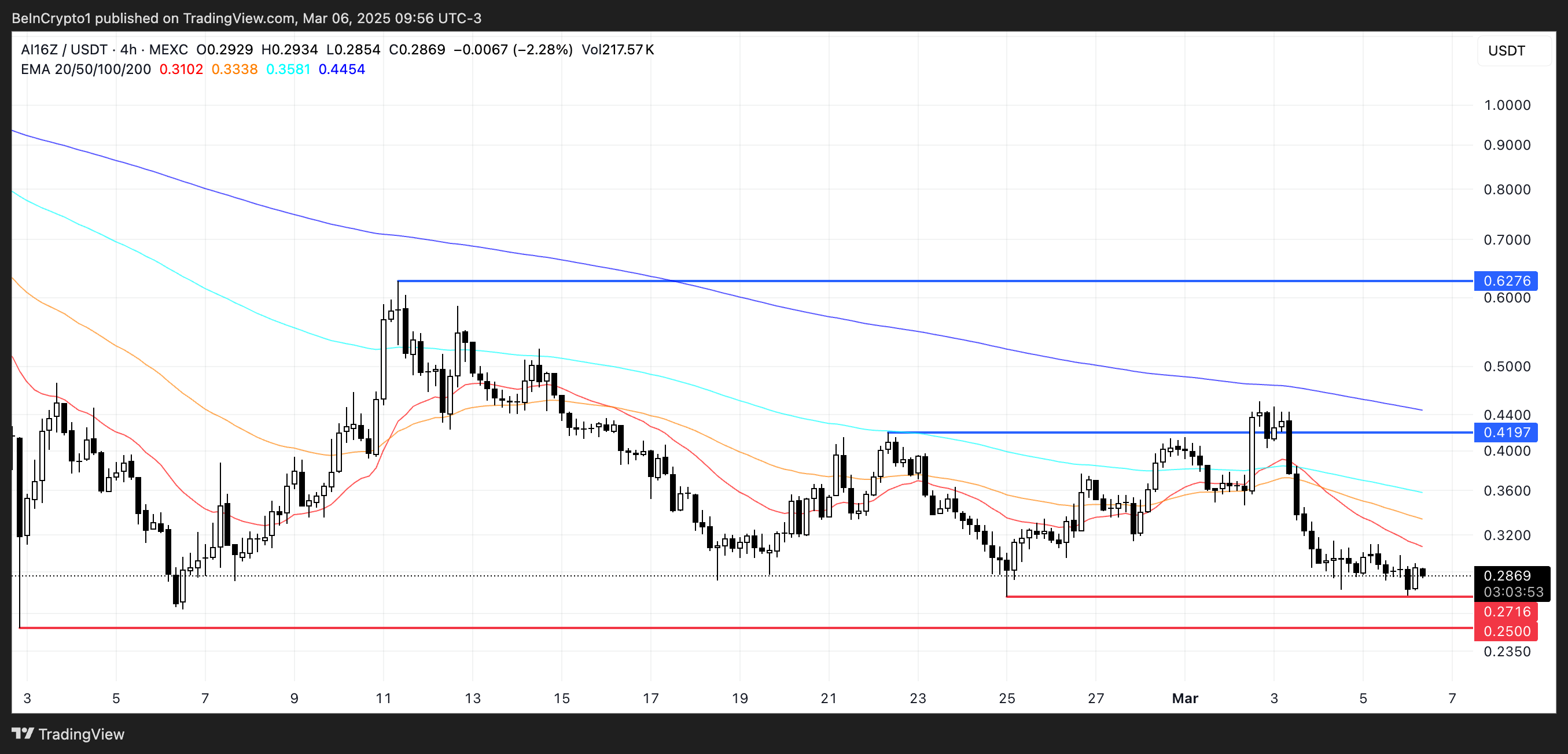
Top 5 memecoins de Solana a tener en cuenta en febrero de 2025
CryptoNewsNet•2025-03-08 07:22
Comprar más
Preguntas frecuentes
¿Cuál es el precio actual de Bonk on ETH?
El precio en tiempo real de Bonk on ETH es €0 por (BONK/EUR) con una capitalización de mercado actual de €0 EUR. El valor de Bonk on ETH sufre fluctuaciones frecuentes debido a la actividad continua 24/7 en el mercado cripto. El precio actual de Bonk on ETH en tiempo real y sus datos históricos están disponibles en Bitget.
¿Cuál es el volumen de trading de 24 horas de Bonk on ETH?
En las últimas 24 horas, el volumen de trading de Bonk on ETH es de €0.00.
¿Cuál es el máximo histórico de Bonk on ETH?
El máximo histórico de Bonk on ETH es €0.{7}5453. Este máximo histórico es el precio más alto de Bonk on ETH desde su lanzamiento.
¿Puedo comprar Bonk on ETH en Bitget?
Sí, Bonk on ETH está disponible actualmente en el exchange centralizado de Bitget. Para obtener instrucciones más detalladas, consulta nuestra útil guía Cómo comprar .
¿Puedo obtener un ingreso estable invirtiendo en Bonk on ETH?
Desde luego, Bitget ofrece un plataforma de trading estratégico, con bots de trading inteligentes para automatizar tus trades y obtener ganancias.
¿Dónde puedo comprar Bonk on ETH con la comisión más baja?
Nos complace anunciar que plataforma de trading estratégico ahora está disponible en el exchange de Bitget. Bitget ofrece comisiones de trading y profundidad líderes en la industria para garantizar inversiones rentables para los traders.
¿Dónde puedo comprar cripto?
Sección de video: verificación rápida, trading rápido
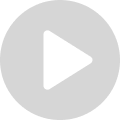
Cómo completar la verificación de identidad en Bitget y protegerte del fraude
1. Inicia sesión en tu cuenta de Bitget.
2. Si eres nuevo en Bitget, mira nuestro tutorial sobre cómo crear una cuenta.
3. Pasa el cursor por encima del ícono de tu perfil, haz clic en "No verificado" y haz clic en "Verificar".
4. Elige tu país o región emisora y el tipo de ID, y sigue las instrucciones.
5. Selecciona "Verificación por teléfono" o "PC" según tus preferencias.
6. Ingresa tus datos, envía una copia de tu ID y tómate una selfie.
7. Envía tu solicitud, ¡y listo! Habrás completado la verificación de identidad.
Las inversiones en criptomoneda, lo que incluye la compra de Bonk on ETH en línea a través de Bitget, están sujetas al riesgo de mercado. Bitget te ofrece formas fáciles y convenientes de comprar Bonk on ETH, y hacemos todo lo posible por informar exhaustivamente a nuestros usuarios sobre cada criptomoneda que ofrecemos en el exchange. No obstante, no somos responsables de los resultados que puedan surgir de tu compra de Bonk on ETH. Ni esta página ni ninguna parte de la información que incluye deben considerarse respaldos de ninguna criptomoneda en particular.
Bitget Insights
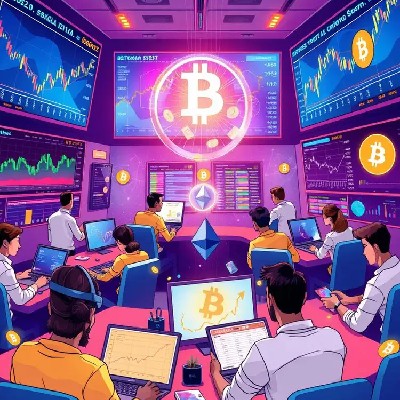
Crypto_inside
8h
What is IQ..🤔🤔??
Intelligence Quotient (IQ) is a score derived from standardized tests designed to measure human intelligence. IQ tests assess various cognitive abilities, such as:
Components of IQ Tests:
1. Verbal Comprehension: Measures ability to understand and use language.
2. Perceptual Reasoning: Assesses ability to reason, form concepts, and solve problems.
3. Working Memory: Evaluates ability to hold and manipulate information in short-term memory.
4. Processing Speed: Measures ability to quickly and accurately process visual information.
IQ Score Interpretation:
1. Average IQ: 85-115 (68% of population)
2. Above Average IQ: 116-130 (16% of population)
3. Gifted IQ: 131-145 (2% of population)
4. Highly Gifted IQ: 146-160 (0.1% of population)
5. Profoundly Gifted IQ: 161-175 (0.01% of population)
Criticisms and Limitations of IQ Tests:
1. Cultural Bias: IQ tests may favor certain cultural or socioeconomic groups.
2. Narrow Scope: IQ tests only measure specific aspects of intelligence.
3. Context-Dependent: IQ scores can be influenced by environmental factors.
4. Oversimplification: IQ scores can oversimplify complex cognitive abilities.
Types of Intelligence:
1. Fluid Intelligence: Ability to reason, think abstractly, and solve problems.
2. Crystallized Intelligence: Ability to use learned knowledge and experience.
3. Emotional Intelligence: Ability to recognize and understand emotions.
Notable Theories and Models:
1. Gardner's Multiple Intelligences: Proposes multiple types of intelligence, such as linguistic, spatial, and bodily-kinesthetic.
2. Sternberg's Triarchic Theory: Suggests three components of intelligence: analytical, creative, and practical.
IQ tests provide a limited snapshot of cognitive abilities and should not be considered the sole measure of intelligence or potential.
Thank you...🙂
$BTC $ETH $SOL $PI $AI $XRP $BGB $BNB $ONDO $DOGE $SHIB $BONK $FLOKI $U2U $WUF $PARTI $WHY $SUNDOG
SUNDOG+0.56%
BTC+2.13%
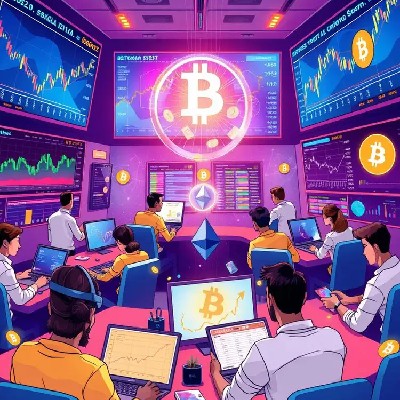
Crypto_inside
8h
Machine learning ❌ Traditional learning. 🧐😵💫
Machine learning and traditional learning are two distinct approaches to learning and problem-solving.
Traditional Learning:
1. Rule-based: Traditional learning involves explicit programming and rule-based systems.
2. Human expertise: Traditional learning relies on human expertise and manual feature engineering.
3. Fixed models: Traditional learning uses fixed models that are not updated automatically.
Machine Learning:
1. Data-driven: Machine learning involves learning from data and improving over time.
2. Algorithmic: Machine learning relies on algorithms that can learn from data and make predictions.
3. Adaptive models: Machine learning uses adaptive models that can update automatically based on new data.
Key Differences:
1. Learning style: Traditional learning is rule-based, while machine learning is data-driven.
2. Scalability: Machine learning can handle large datasets and complex problems, while traditional learning is limited by human expertise.
3. Accuracy: Machine learning can achieve higher accuracy than traditional learning, especially in complex domains.
Advantages of Machine Learning:
1. Improved accuracy: Machine learning can achieve higher accuracy than traditional learning.
2. Increased efficiency: Machine learning can automate many tasks, freeing up human experts for more complex tasks.
3. Scalability: Machine learning can handle large datasets and complex problems.
Disadvantages of Machine Learning:
1. Data quality: Machine learning requires high-quality data to learn effectively.
2. Interpretability: Machine learning models can be difficult to interpret and understand.
3. Bias: Machine learning models can perpetuate biases present in the training data.
When to Use Machine Learning:
1. Complex problems: Machine learning is well-suited for complex problems that require pattern recognition and prediction.
2. Large datasets: Machine learning can handle large datasets and identify trends and patterns.
3. Automating tasks: Machine learning can automate many tasks, freeing up human experts for more complex tasks.
When to Use Traditional Learning:
1. Simple problems: Traditional learning is well-suited for simple problems that require explicit programming and rule-based systems.
2. Small datasets: Traditional learning is suitable for small datasets where machine learning may not be effective.
3. Human expertise: Traditional learning relies on human expertise and manual feature engineering, making it suitable for domains where human expertise is essential.
Thank you...🙂
$BTC $ETH $SOL $PI $AI $XAI $BGB $BNB $DOGE $DOGS $SHIB $BONK $MEME $XRP $ADA $U2U $WUF $PARTI $WHY
BTC+2.13%
BGB+2.22%
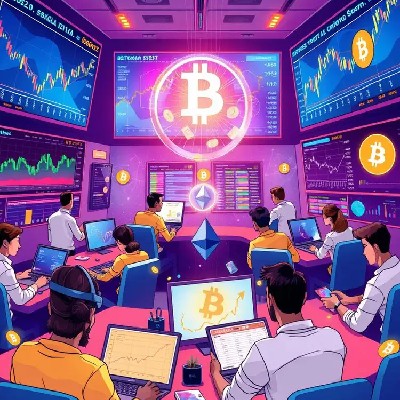
Crypto_inside
8h
What is Q-learning...🤔🤔??
Q-learning is a type of reinforcement learning algorithm used in machine learning and artificial intelligence. It's a model-free, off-policy learning algorithm that helps agents learn to make decisions in complex, uncertain environments.
Key Components:
1. Agent: The decision-maker that interacts with the environment.
2. Environment: The external system with which the agent interacts.
3. Actions: The decisions made by the agent.
4. Rewards: The feedback received by the agent for its actions.
5. Q-function: A mapping from states and actions to expected rewards.
How Q-learning Works:
1. Initialization: The agent starts with an arbitrary Q-function.
2. Exploration: The agent selects an action and observes the resulting state and reward.
3. Update: The agent updates its Q-function based on the observed reward and the expected reward for the next state.
4. Exploitation: The agent chooses the action with the highest Q-value for the current state.
Advantages:
1. Simple to implement: Q-learning is a straightforward algorithm to understand and code.
2. Effective in complex environments: Q-learning can handle complex, dynamic environments with many states and actions.
Disadvantages:
1. Slow convergence: Q-learning can require many iterations to converge to an optimal policy.
2. Sensitive to hyperparameters: The performance of Q-learning is highly dependent on the choice of hyperparameters.
Q-learning is a powerful algorithm for reinforcement learning, but it can be challenging to tune and may not always converge to an optimal solution.
Thank you...🙂
$BTC $ETH $SOL $PI $AI $XAI $XRP $BGB $BNB $DOGE $DOGS $SHIB $BONK $FLOKI $U2U $WUF $WHY $SUNDOG $COQ $PEPE
SUNDOG+0.56%
BTC+2.13%
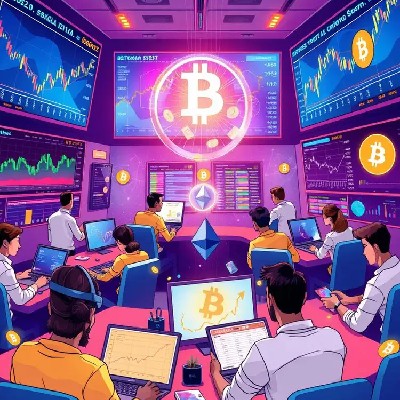
Crypto_inside
8h
What is Machine learning..🤔🤔??
Machine learning is a subset of artificial intelligence (AI) that involves training algorithms to learn from data and make predictions, decisions, or recommendations without being explicitly programmed.
Key Characteristics:
1. Learning from data: Machine learning algorithms learn patterns and relationships in data.
2. Improving over time: Machine learning models improve their performance as they receive more data.
3. Making predictions or decisions: Machine learning models make predictions, decisions, or recommendations based on the learned patterns.
Types of Machine Learning:
1. Supervised Learning: The algorithm learns from labeled data to make predictions.
2. Unsupervised Learning: The algorithm learns from unlabeled data to identify patterns.
3. Reinforcement Learning: The algorithm learns through trial and error to achieve a goal.
4. Semi-supervised Learning: The algorithm learns from a combination of labeled and unlabeled data.
5. Deep Learning: A subset of machine learning that uses neural networks with multiple layers.
Machine Learning Applications:
1. Image Recognition: Image classification, object detection, and facial recognition.
2. Natural Language Processing (NLP): Text classification, sentiment analysis, and language translation.
3. Speech Recognition: Speech-to-text and voice recognition.
4. Predictive Analytics: Forecasting, regression, and decision-making.
5. Recommendation Systems: Personalized product recommendations.
Machine Learning Algorithms:
1. Linear Regression: Linear models for regression tasks.
2. Decision Trees: Tree-based models for classification and regression.
3. Random Forest: Ensemble learning for classification and regression.
4. Support Vector Machines (SVMs): Linear and non-linear models for classification and regression.
5. Neural Networks: Deep learning models for complex tasks.
Machine Learning Tools and Frameworks:
1. TensorFlow: Open-source deep learning framework.
2. PyTorch: Open-source deep learning framework.
3. Scikit-learn: Open-source machine learning library.
4. Keras: High-level neural networks API.
Machine learning has numerous applications across industries, including healthcare, finance, marketing, and more. Its ability to learn from data and improve over time makes it a powerful tool for solving complex problems.
Thank you...🙂
$BTC $ETH $SOL $PI $AI $XAI $BGB $BNB $DOGE $SHIB $FLOKI $BONK $U2U $WUF $WHY $SUNDOG $PARTI $XRP
SUNDOG+0.56%
BTC+2.13%
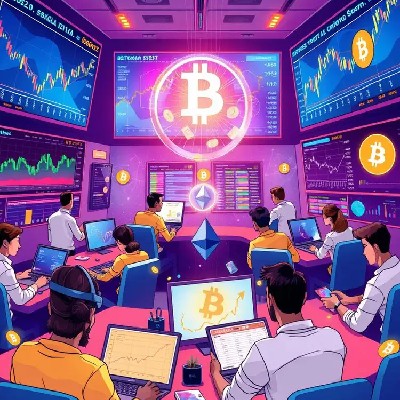
Crypto_inside
21h
Price action ❌ Technical analysis. 🧐😵💫
Price action and technical analysis are two related but distinct concepts in trading and investing.
Price Action:
1. Focuses on raw price data: Price action involves analyzing the price movement of a security over time.
2. No indicators or overlays: Price action traders rely solely on the price chart, without using technical indicators or overlays.
3. Emphasis on market structure: Price action traders study the structure of the market, including trends, reversals, and breakouts.
Technical Analysis:
1. Uses indicators and overlays: Technical analysis involves using various indicators and overlays, such as moving averages, RSI, and Bollinger Bands, to analyze price data.
2. *Focuses on patterns and trends*: Technical analysis identifies patterns and trends in price data, using indicators and overlays to confirm or contradict the analysis.
3. *Includes various methods*: Technical analysis encompasses various methods, including chart patterns, trend analysis, and momentum analysis.
Key Differences:
1. Use of indicators: Price action traders do not use indicators, while technical analysts rely heavily on them.
2. Focus: Price action focuses on raw price data and market structure, while technical analysis focuses on patterns, trends, and indicators.
3. Approach: Price action trading is often more discretionary and subjective, while technical analysis can be more systematic and rule-based.
Similarities:
1. Both analyze price data: Both price action and technical analysis involve analyzing price data to make trading decisions.
2. Both aim to identify trends and patterns: Both approaches aim to identify trends, patterns, and other market structures to inform trading decisions.
3. Both require skill and experience: Both price action and technical analysis require skill, experience, and continuous learning to master.
In summary, while price action and technical analysis share some similarities, they differ in their approach, focus, and use of indicators. Price action traders rely solely on raw price data and market structure, while technical analysts use indicators and overlays to identify patterns and trends.
Thank you...🙂
$BTC $ETH $SOL $PI $XRP $ADA $AI $DOGE $SHIB $FLOKI $BONK $BGB $BNB $U2U $PARTI $WUF $WHY $SUNDOG $DOGS $GEEK
SUNDOG+0.56%
BTC+2.13%
Activos relacionados
Criptomonedas populares
Una selección de las 8 criptomonedas principales por capitalización de mercado.
Agregada recientemente
Las criptomonedas agregadas más recientemente.
Capitalización de mercado comparable
Entre todos los activos de Bitget, estos 8 son los más cercanos a Bonk on ETH en capitalización de mercado.
